Data Science Applied In Business
In the past decade, there has been an explosion in the application of data science outside of academic realms. The use of general, statistical, predictive machine learning models has achieved high success rates across multiple occupations including finance, marketing, sales, and engineering, as well as multiple industries including entertainment, online and store front retail, transportation, service and hospitality, healthcare, insurance, manufacturing and many others. The applications of data science seem to be nearly endless in today’s modern landscape, with each company jockeying for position in the new data and insights economy. Yet, what if I told you that companies may be achieving only a third of the value they could be getting with the use of data science for their companies? I know, it sounds almost fantastical given how much success has already been achieved using data science. However, many opportunities for value generation may be getting over looked because data scientists and statisticians are not traditionally trained to answer some of the questions companies in industry care about.
Most of the technical data science analysis done today is either classification (labeling with discrete values), regression (labeling with a number), or pattern recognition. These forms of analysis answer the business questions ‘can I understand what is going on’ and ‘can I predict what will happen next’. Examples of questions are ‘can I predict which customers will churn?’, ‘can I forecast my next quarter revenue?’, ‘can I predict products customers are interested in?’, ‘are there important customer activity patterns?’, etc… These are extremely valuable questions to companies that can be answered by data science. In fact, answering these questions is what has caused the explosion in interest in applying data science in business applications. However, most companies have two other major categories of important questions that are being totally ignored. Namely, once a problem has been identified or predicted, can we determine what’s causing it? Furthermore, can we take action to resolve or prevent the problem?
I start this article discussing why most data driven companies aren’t as data driven as they think they are. I then introduce the idea of the 3 categories of questions companies care about the most (The Big 3), discuss why data scientists have been missing these opportunities. I then outline how data scientists and companies can partner to answer these questions.
Why Even Advanced Tech Companies Aren’t as Data Driven As They Think They Are.
Many companies want to become more ‘data driven’, and to generate more ‘prescriptive insights’. They want to use data to make effective decisions about their business plans, operations, products and services. The current idea of being ‘data driven’ and ‘prescriptive insights’ in the industry today seems to be defined as using trends or descriptive statistics about about data to try to make informed business decisions. This is the most basic form of being data driven. Some companies, particularly the more advanced technology companies go a step further and use predictive machine learning models and more advanced statistical inference and analysis methods to generate more advanced descriptive numbers. But that’s just it. These numbers, even those generated by predictive machine learning models, are just descriptive (those with a statistical background must forgive me for the overloaded use of the term ‘descriptive’). They may be descriptive in different ways, such as machine learning generating a predicted number about something that may happen in the future, while a descriptive statistic indicates what is happening in the present, but these methods ultimately focus on producing a number. To take action to bring about a desired change in an environment requires more than a number. It’s not enough to predict a metric of interest. Businesses want to use numbers to make decisions. In other words, businesses want causal stories. They want to know why a metric is the way it is, and how their actions can move that metric in a desired direction. The problem is that classic statistics and data science falls short in pursuit of answers to these questions.
Take the example diagram shown in figure 1 below. Figure 1 shows a very common business problem of predicting the risk of a customer churning. For this problem, a data scientist may gather many pieces of data (features) about a customer and then build a predictive model. Once a model is developed, it is deployed as a continually running insight service, and integrated into a business process. In this case, let’s say we have a renewal manager that wants to use these insights. The business process is as follows. First, the automated insight service that was deployed gathers data about the customer. It then passes that data to the predictive model. The predictive model then outputs a predicted risk of churn number. This number is then passed to the renewal manager. The renewal manager then uses their gut intuition to determine what action to take to reduce the risk of churn. This all seems straightforward enough. However, we’ve broken the chain of being data driven. How is that you ask? Well, our data driven business process stopped at the point of generating our churn risk number. We simply gave our churn risk number to a human, and they used their gut intuition to make a decision. This isn’t data driven decision making, this is gut driven decision making. It’s a subtle thing to notice, so don’t feel too bad if you didn’t see it at first. In fact, most people don’t recognize this subtlety. That’s because it’s so natural these days to think that getting a number to a human is how making ‘data driven decisions’ works. The subtlety exists because we are not using data and statistical methods to evaluate the impact of actions the human can take on the metric they care about. A human sees a number or a graph, and then *decides* to take *action*. This implies they have an idea about how their *action* will *effect* the number or graph that they see. Thus, they are making a cause and effect judgement about their decision making and their actions. Yet, they aren’t using any sort of mathematical methods for evaluating their options. They are simply using their personal judgement to make a decision. What can end up happening in this case is that a human may see a number, make a decision, and end up making that number worse.
Let’s take the churn risk example again. Let’s say the customer is 70% likely to churn and that they were likely to churn because their experience with the service was poor, but assume that the renewal manager doesn’t know this (this too is actually a cause and effect statement). Let’s also say that a renewal manager sends a specially crafted renewal email to this customer in an attempt to reduce the likelihood of churn. That seems like a reasonable action to take, right? However, this customer receives the email, and is reminded of how bad their experience was, and is now even more annoyed with our company. Suddenly the likelihood to churn increases to 90% for this customer. If we had taken no action, or possibly a different action (say connecting them with digital support resources) then we would have been better off. But without an analysis of cause and effect, and without systems that can analyze our actions and prescribe the best ones to take, we are gambling with the metrics we care about.
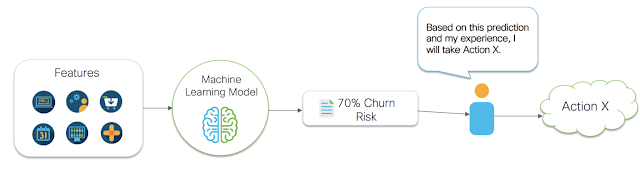
Figure 1
So how can we attempt to solve this problem? We need to incorporate mathematical models and measurement into the business process after the number is generated. We need to collect data on what actions are being taken, measure their relationship with the metrics we care about, and then optimize over our actions using causal inference models and AI systems. Figure 2 below shows how we can insert an AI system into the business process to help track, measure, and optimize the actions our company is taking. Using a combination of mathematical analysis methods, we can begin to optimize the entire process using data science end to end. The stages of this process can be abstracted and generalized as answering 3 categories of questions companies care about. Those 3 categories are described in the next section.
Comparing Machine Learning to Causal Analysis (Inference)
To get a better understanding of what machine learning does and where it falls short, we introduce figure 3 and figure 4 below. Figure 3 and Figure 4 both describe the problem space of understanding cancer. Machine learning can be used to do things like predict whether or not a patient will get cancer given characteristics that have been measured about them. Figure 3 shows this by assigning directed arrows from independent variables to the dependent variable (in this case cancer). These links are associative by their construction. The main point is that machine learning focuses on numbers and the accurate production of a number. This can in many cases be enough to gain a significant amount of value. For example, predicting the path of a hurricane has value on it’s own. There exists no confusion about what should be done given the prediction. If you are in the predicted path of the hurricane, the action is clearly to get out of the way. Sometimes however, we want to know why something is happening. Many times we want to play ‘what-if’ games. What if the patient stopped smoking? What if the patient had less peer pressure? To answer these questions, we need to perform a causal analysis.
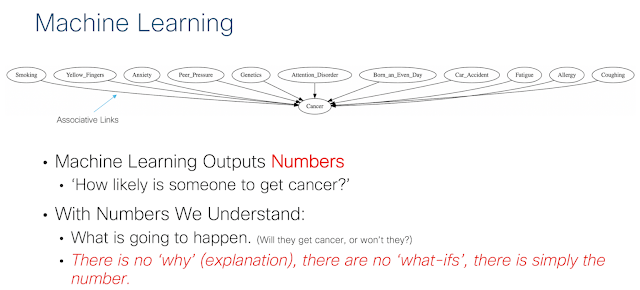
Figure 3
Figure 4 below shows a visual example of what causal analysis provides. Causal analysis outputs stories, not just numbers. The diagram shows the directed causal links between all variables in an environment. For example, given this diagram anxiety causes smoking. Causal stories are important any time we or our business stakeholders want to take action to improve the environment. The causal story allows us to quantify cause and effect relationships, play what-if scenarios, and perform root-cause analysis. Machine learning falls short of being able to do this because these all require a modeling of cause and effect relationships.
What Are the Big 3?
Figure 5
Figure 5 above describes what ‘The Big 3’ questions companies care about are. The big 3 questions seem fairly obvious. In fact, these questions are at the foundation of most of problem solving in the real world. Yet, almost all data science in industry today revolves around answering only the first question. What most data scientists understand as supervised, unsupervised, and semi-supervised learning revolves around answering what is happening or what will happen. Even with something like a product recommendation system (which you might believe prescribes something because of the term ‘recommend’), we only know what products a customer is interested in (thus it’s only an indication of interest, not a reason for interest). We don’t know the most effective way to act on that information. Should we send an ad? Should we call them? Do certain engagements with them cause a decrease their chances of purchase? To answer what is *causing* something to happen, we need to rely on foundational work in the area of Causal Inference developed by researchers like Ronald Fisher, Jerzy Neyman, Judea Pearl, Donald B. Rubin, Paul Holland, and many others. Once we understand what is causing a metric we care about, we can at least begin to think intelligently about the actions we can take to change those metrics. This is where the third question mentioned in figure 3 above comes in. To answer this question we can rely on a wide variety of techniques that have been developed including causal inference for the cause and effect relationship between actions and the metrics they are supposed to affect, statistical decision theory, decision support systems, control systems, reinforcement learning, and game theory.

Figure 6
Figure 6 above breaks down some of the methods in a more technical way. The methodology column outlines the major methods, fields, and approaches that can be in general used each of the big 3 questions in turn. The algorithms column lists some specific algorithms that may be applied to answer each of the big 3 questions. While some of these algorithms should be familiar to the average data scientist (deep neural networks, random forests, etc.), others are maybe only known in passing (multi-armed bandits, reinforcement learning, etc). Still more algorithms are likely to be totally new to some data scientists (Difference in Differences, Propensity Score Matching, etc). The main paper delves into each question and the important technical details of the methods used to answer each question. It’s very important to understand these methods, particularly for performing causal analysis and optimizing actions. These methods are highly nuanced, with many difference kinds of assumptions. Naively applying these methods without understanding their limitations and assumptions will almost certainly lead to incorrect conclusions.
Example Use Case for Renewals.
A well known question, in which we have applied the big 3 methodology, is for understanding Cisco product and service renewals. Understanding and predicting renewals is a prime example of how many companies are attempting to get value through data science. The problem is also typically referred to as predicting churn, churn risk prediction, predicting attrition. Focusing on renewals is also useful for demonstration purposes because most of the data science applied to problems of this kind fall short of providing full value. That’s because renewals is a problem where providing a number is not the goal. Simply providing likelihood of a customer to renew is not enough. The company wants to **do** something about this. The company wants to take action to cause an increase in the likelihood to renew. For this, and any other time where the goal is to **do** something, we rely on causal inference and methods for optimizing actions.
Question 1: What is happening or will happen?
As we’ve already stated, the main question that is typically posed to a team of data scientists is ‘Can we accurately predict which customers will renew and which ones won’t?’ While this is a primary question asked by the business, there are many other questions that fall into the area of prediction and pattern mining including,
1. How much revenue can we expect from renewals? What does the distribution look like?
2. What’s the upper/lower bound on the expected revenue predicted by the models?
3. What are the similar attributes among customers likely to churn versus not churn?
4. What are the descriptive statistics for customers likely to churn vs not churn collectively, in each label grouping, and in each unsupervised grouping?
Each of the above questions can be answered systematically by framing them as problems either in prediction or pattern mining, and by using the wide variety of mathematical methods found in the referenced materials in the main paper here. These are the questions and methods data scientists are most familiar, and will most commonly be answered for a business.
Question 2: Why is this happening or going to happen?
Given this first question, the immediate next question is why. Why are customers likely or not likely to churn? For each question that we can build a model for, we can also perform a causal analysis. Thus, we can already potentially double the value that a data science project returns by simply adding on a causal analysis to each predictive model built. It’s important to bring up again that this question is so important that most data scientists are either answering it incorrectly, or are misrepresenting the information from statistical associations.
Specifically, when a data scientist is asked the question of why a customer is likely to churn, they almost exclusively turn to feature importance and local models such as LIME, SHAP, and others. These methods for describing the reason for a prediction are almost always incorrect because there is a disconnect between what the business stakeholder is asking for and what the data scientist is providing because of two different interpretations of the term ‘why’. Technically, one can argue that feature importance measures what features are important to ‘why’ a model makes a prediction, and this would be correct. However, a business stakeholder usually wants to know ‘what is causing the metric itself’ and not ‘what is causing the metric prediction’. The business stakeholder wants to know the causal mechanisms for why a metric is a particular number. This is something that feature importance absolutely does not answer. The stakeholder wants to use the understanding of the causal mechanisms to take an action to change the prediction to be more in their favor. This requires a causal analysis. However, most data scientists simply take the features with highest measured importance and present them to the stakeholder as though they are the answer to their causal question. This is objectively wrong, yet is time and again presented to stakeholders by seasoned statisticians and data scientists.
The issue is compounded by the further confusion added by discussions around ‘interpretable models’ and by the descriptions of feature importance analysis. LIME describes it’s package as ‘explaining what machine learning classifiers (or models) are doing’. While still a technically correct statement, these methods are being used to incorrectly answer causal questions, leading stakeholders to take actions that may have the opposite effect of what they intended.
While we’ve outlined the main causal question, there are a number of questions that can also be asked, and corresponding analysis that can be performed including,
1. How are variables correlated with each other and the churn label? (A non-causal question)
2. What are the important features for prediction in a model in general? (A non-causal question)
3. What are the most important features for prediction for an individual? Do groupings of customers with locally similar relationships exist? (A non-causal question)
4. What are the possible confounding variables? (A causal question)
5. After controlling for confounding variables, how do the predictions change? (A non-causal question benefiting from causal methods)
6. What does the causal bayes net structure look like? What are all of the reasonable structures? (A causal question)
7. What are the causal effect estimates between variables? What about between variables and the class label? (A causal question).
Many of these questions can be answered in whole or in part by a thorough causal analysis using the methods we outlined in the corresponding causal inference section of the main paper here, and further multiply the value returned by a particular data science project.
Question 3: How can we take action to make the metrics improve?
The third question to answer is ‘what actions can a stakeholder take to prevent churn?’ This is ultimately the most valueable of the three questions. The first two question set the context for who to focus on and where to focus efforts. Answering this question provides stakeholders with a directed and statistically valid means to improve the metrics they care about given complex environments. While still challenging given the methods available today (and presented in the section on intelligent action), it provides one of the greatest value opportunities. Some other questions that can be answered related to intelligent action that stakeholders may be interested in include,
1. What variables are likely to reduce churn risk if our actions could influence them?
2. What actions have the strongest impact on the variables that are likely to influence churn risk, or to reduce churn risk directly?
3. What are the important pieces of contextual information relevant for taking an action?
4. What are the new actions that should be developed and tested in an attempt to influence churn risk?
5. What actions are counter-productive or negatively impact churn risk?
6. What does the diminishing marginal utility of an action look like? At what point should an action no longer be taken?
The right method to use for prescribing intelligent action depends largely on the problem and the environment. If the environment is complex, the risks high, and there is not much chance for an automated system to be implemented, then methods from causal inference, decision theory, influence diagrams, and game theory based analysis are good candidates. However, if a problem and stakeholder are open to the use of an automated agent to learn and prescribe intelligent actions, then reinforcement learning may be a good choice. While possibly the most valuable of the big three questions to answer, it also exists as one of the most challenging. There still many open research questions related to answering this question, but the value proposition means that it’s likely an area that will see increased industry investment in the coming years.
How We Are Improving CX By Using Data Science to Answer the Big 3 at Cisco.
Like many other companies, Cisco has many models for answering the first of the big 3 questions. The digital lifecycle journey data science team has many predictive models for understanding Cisco’s customers. This includes analysis of customer purchasing behavior, digital activity, telemetry, support interaction, and renewal activity using a wide variety of machine learning based algorithms. We also apply the latest and greatest forms of advanced statistical and deep learning based supervised learning methods for understanding and predicting the expected behavior of our customers, their interactions with Cisco, and their interactions with Cisco products and services. We go a step further in this area by attempting to quantify and predict metrics valuable to both Cisco and Cisco’s customers. For example, we predict metrics like how a customer is going to keep progressing through the expected engagement with their product over the next several days to next several weeks. This is just one of the many metrics we are trying to understand about the Cisco customer experience. Others include customer satisfaction, customer health, customer ROI, renewal metrics, and many others. These metrics allow us to understand where there may be issues with our journey so that we can start trying to apply data science methods to answer the ‘why’ and ‘intelligent action’ questions we’ve previously mentioned.
We are also using causality to attempt to understand the Cisco’s customer experience, and what causes a good or bad customer experience. We go a step further by trying to complete the causal chain of reasoning to quantify how a customer experience causes Cisco’s business metrics to rise and fall. For example, we’ve used causal inference methods to measure the cause and effect aspects of customer behavior, product utilization, and digital engagements on a customer’s likelihood to renew Cisco services. Using causal inference, we are gaining deeper insights into what is causing our customers and Cisco to succeed or fail, and are using that information to guide our strategy for maximizing the customer experience.
Finally, to answer the third of the big three questions, we are employing causality, statistical decision theory, intelligent agent theory, and reinforcement learning to gain visibility to the impact our activities have on helping our customers and improving Cisco’s business metrics, and to learn to prescribe optimal actions over time to maximize these metrics. We have developed intelligent action systems that we working to integrate with our digital email engagements journeys to optimize our interactions with customers to help them achieve a return on investment. We are, in general, applying this advanced intelligent agent system to quantify the impact of our digital interactions, and to prescribe the right digital customer engagements to have, with the most effective content, at the right time, in the right order, personalized to each and every individual customer.
Why Many Data Scientists Don’t Know the Big 3, or How to Answer Them.
Those learned readers experienced with data science may be asking themselves, ‘is anything new being said here’? It’s true that no new technical algorithm, mathematical analysis, or in depth proof is being presented. I’m not presenting some new mathematical modeling method, or some novel comparison of existing methods. What is new is how I’m framing the problems for data science in industry, and the existing methodologies that can start to solve those problems. Causal inference has been used heavily in medicine for observational studies where controlled trials aren’t feasible, and for things like adverse drug effect discovery. However, Causal Inference hasn’t received wide spread application in areas outside of the medical, economic, or social science fields yet. The idea of prescribed actions is also something that isn’t totally new. Prescribed actions can be thought of as just a restatement of the field of control systems, decision analysis, and intelligent agent theory. However, the utilization of these methods for completing the end-to-end data driven methodology for business hasn’t received wide spread application in industry applications. Why is this? Why aren’t data scientists and businesses working together to frame all of their problems this way?
There could be a couple of reasons for this. The most obvious answer is that most data scientists are trained to answer the first of the big 3 questions. Most data scientists and statisticians are trained on statistical inference, classification, regression, and general unsupervised learning methods like clustering and anomaly detection. Statistical methods like causal inference aren’t widely known, and are therefore not widely taught. Register with any online course, university, or other platform for learning about data science and machine learning and you’ll be hard pressed to find discussions about identifying causal patterns in data sets. The same goes for the ideas of intelligent agents, control systems, and reinforcement learning to a lesser degree. These methods tend to be relegated to domains that have simulators and a tolerance for failure. Thankfully there is less of a gap for these methods. They typically are given their own special courses in either machine learning, electronics, and signals and systems processing curriculums.
Another possible explanation may be that many data scientists in industry tend to be enamored with the latest and greatest popular algorithm or methodology. As math and tech nerds we become enamored with the technical intricacies of how things work, particularly mathematical algorithms and methodologies. We tend to develop models and then go looking for solutions rather than the other way around, potentially blinding us to the methods in data science that can provide business value time and time again.
Yet another explanation may be that many data scientists are not well versed enough in statistics and the statistical literature. Many data scientists are asked questions about how a predictive model produced a number. For example, in our churn risk problem, renewal managers typically want to know why someone is at risk. The average data scientist hears this, and then uses methods like feature importance and more interpretable models to answer this question. However this doesn’t really answer the actual question being asked. The data scientist provides what might be important associations between model inputs and the predicted metric, but this doesn’t provide the information the renewal manager wants. They want to know about information they can act on, which requires cause and effect analysis. This is a classic case of ‘correlation is not causation’ that everyone seems to know but can still trip up even statistically minded data scientists. It’s such an issue that many companies I’ve talked with that claim to provide ‘next best actions’ are statistically invalid (mainly because they use feature importance and sensitivity analysis type methods instead of understanding basic counter factual analysis and confounding variables).
Moving forward the data science community operating in industry domains will become more aware of the big 3 questions and the analysis methods that can be performed to answer them. Companies that can quickly realize value from answering these questions using data science will be at the head of the pack in the emerging data science and insights economy. Companies that focus on answering all of the big 3 questions will have a distinct competitive advantage, and will have transformed themselves to be truly data driven.