You speak to a customer representative, and they tell you one thing.
You log into your digital account and see another.
You receive an email from the same company that tells an entirely different story.
At Cisco, we have been working to identify these friction points and evaluating how we can orchestrate a more seamless experience—transforming the customer, partner, and seller experience to be prescriptive, helpful – and, most importantly, simple. This is not an easy task when working in the complexity of environments, technologies, and client spaces that Cisco does business in, but it is not insurmountable.
We just closed out a year-long pilot of an industry-leading orchestration vendor, and by all measures – it failed. In The Lean Startup Eric Ries writes, “if you cannot fail, you cannot learn.” I fully subscribe to this perspective. If you are not willing to experiment, to try, to fail, and to evaluate your learnings, you only repeat what you know. You do not grow. You do not innovate. You need to be willing to dare to fail, and if you do, to try to fail forward.
So, while we did not renew the contract, we did continue down our orchestration journey equipped with a year’s worth of learnings and newly refined direction on how to tackle our initiatives.
Our Digital Orchestration Goals
We started our pilot with four key orchestration use cases:
1. Seamlessly connect prescriptive actions across channels to our sellers, partners, and customers.
2. Pause and resume a digital email journey based on triggers from other channels.
3. Connect analytics across the multichannel customer journey.
4. Easily integrate data science to branch and personalize the customer journey.
Let’s dive a bit deeper into each. We’ll look at the use case, the challenges we encountered, and the steps forward we are taking.
Use Case #1: Seamlessly connect prescriptive actions across channels to our sellers, partners, and customers.
Today we process and deliver business-defined prescriptive actions to our customer success representatives and partners when we have digitally identified adoption barriers in our customer’s deployment and usage of our SaaS products.
In our legacy state, we were executing a series of complex SQL queries in Salesforce Marketing Cloud’s Automation Studio to join multiple data sets and output the specific actions a customer needs. Then, using Marketing Cloud Connect, we wrote the output to the task object in Salesforce CRM to generate actions in a customer success agent’s queue. After this action is written to the task object, we picked up the log in Snowflake, applied additional filtering logic and wrote actions to our Cisco partner portal – Lifecycle Advantage, which is hosted on AWS.
There are several key issues with this workflow:
◉ Salesforce Marketing Cloud is not meant to be used as an ETL platform; we were already encountering time out issues.
◉ The partner actions were dependent on the seller processing, so it introduced complexity if we ever wanted to pause one workflow while maintaining the other.
◉ The development process was complex, and it was difficult to introduce new recommended actions or to layer on additional channels.
◉ There was no feedback loop between channels, so it was not possible for a customer success representative to see if a partner had taken action or not, and vice versa.
Thus, we brought in an orchestration platform – a place where we can connect multiple data sources through APIs, centralize processing logic, and write the output to activation channels. Pretty quickly in our implementation, though, we encountered challenges with the orchestration platform.
The Challenges
◉ The complexity of the joins in our queries could not be supported by the orchestration platform, so we had to preprocess the actions before they entered the platform and then they could be routed to their respective activation channels. This was our first pivot. In our technical analysis of the platform, the vendor assured us that our queries could be supported in the platform, but in actual practice, that proved woefully inaccurate. So, we migrated the most complex processing to Google Cloud Platform (GCP) and only left simple logic in the orchestration platform to identify which action a customer required and write that to the correct activation channel.
◉ The user interface abstracted parts of the code creating dependencies on an external vendor. We spent considerable time trying to decipher what went wrong via trial and error without access to proper logs.
◉ The connectors were highly specific and required vendor support to setup, modify, and troubleshoot.
Our Next Step Forward
These three challenges forced us to think differently. Our goal was to centralize processing logic and connect to data sources as well as activation channels. We were already leveraging GCP for preprocessing, so we migrated the remainder of the queries to GCP. In order to solve for our need to manage APIs to enable data consumption and channel activation, we turned to Mulesoft. The combination of GCP and Mulesoft helped us achieve our first orchestration goal while giving us full visibility to the end-to-end process for implementation and support.
Orchestration Architecture
Use Case #2: Pause and resume a digital email journey based on triggers from other channels.
We focused on attempting to pause an email journey in a Marketing Automation Platform (Salesforce Marketing Cloud or Eloqua) if a customer had a mid-to-high severity Technical Assistance Center (TAC) Case open for that product.
Again, we set out to do this using the orchestration platform. In this scenario, we needed to pause multiple digital journeys from a single set of processing logic in the platform.
The Challenge
We did determine that we could send the pause/resume trigger from the orchestration platform, but it required setting up a one-to-one match of journey canvases in the orchestration platform to journeys that we might want to pause in the marketing automation platform. The use of the orchestration platform actually introduced more complexity to the workflow than managing ourselves.
Our Next Step Forward
Again, we looked at the known challenge and the tools in our toolbox. We determined that if we set up the processing logic in GCP, we could evaluate all journeys from a single query and send the pause trigger to all relevant canvases in the marketing automation platform – a much more scalable structure to support.
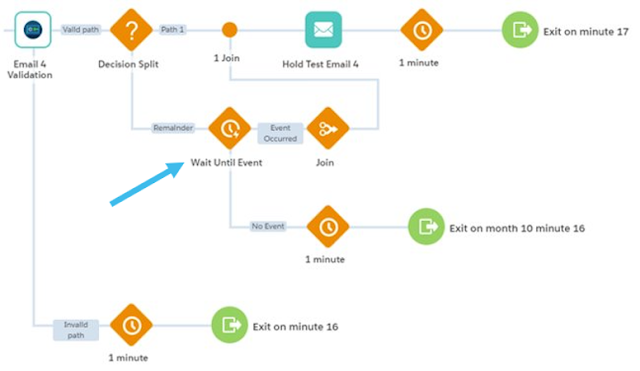
Sample of Wait Until Event used in Journey Builder

Wait Until API Configuration
Another strike against the platform, but another victory in forcing a new way of thinking about a problem and finding a solution we could support with our existing tech stack. We also expect the methodology we established to be leveraged for other types of decisioning such as journey prioritization, journey acceleration, or pausing a journey when an adoption barrier is identified and a recommended action intervention is initiated.
Use Case #3: Connect analytics across the multichannel customer journey.
We execute journeys across multiple channels. For instance, we may send a renewal notification email series, show a personalized renewal banner on Cisco.com for users of that company with an upcoming renewal, and enable a self-service renewal process on renew.cisco.com. We collect and analyze metrics for each channel, but it is difficult to show how a customer or account interacted with each digital entity across their entire experience.
Orchestration platforms offer analytics views that display Sankey diagrams so journey strategists can visually review how customers engage across channels to evaluate drop off points or particularly critical engagements for optimization opportunities.
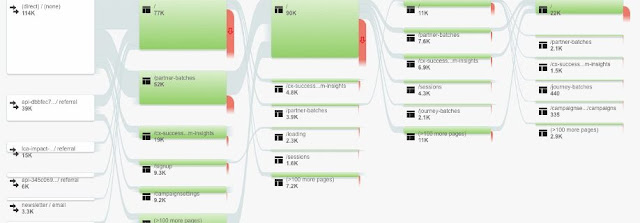
Sample of a Sankey Diagram
The Challenge
◉ As we set out to do this, we learned the largest blocker to unifying this data is not really a challenge an orchestration platform innately solves just through executing the campaigns through their platform. The largest blocker is that each channel uses different identifiers for the customer. Email journeys use email address, web personalization uses cookies associated at an account level, and the e-commerce experience uses user ID login. The root of this issue is the lack of a unique identifier that can be threaded across channels.
◉ Additionally, we discovered that our analytics and metrics team had existing gaps in attribution reporting for sites behind SSO login, such as renew.cisco.com.
◉ Finally, since many teams at Cisco are driving web traffic to Cisco.com, we saw a large inconsistency with how different teams were tagging (and not tagging) their respective web campaigns. To be able to achieve a true view of the customer journey end to end, we would need to adopt a common language for tagging and tracking our campaigns across business units at Cisco.
Our Next Step Forward
Our team began the process to adopt the same tagging and tracking hierarchy and system that our marketing organization uses for their campaigns. This will allow our teams to bridge the gap between a customer’s pre-purchase and post-purchase journeys at Cisco—enabling a more cohesive customer experience.
Next, we needed to tackle the data threading. Here we identified what mapping tables existed (and where) to be able to map different campaign data to a single data hierarchy. For this particular example for renewals, we needed to tackle three different data hierarchies:
1. Party ID associated with a unique physical location for a customer who has purchased from Cisco
2. Web cookie ID
3. Cisco login ID
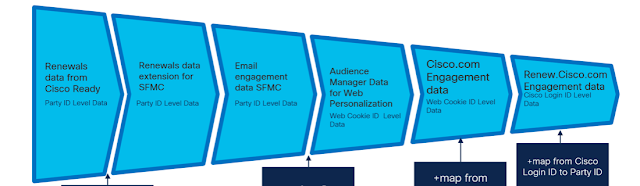
Data mapping exercise for Customer Journey Analytics
With the introduction of consistent, cross Cisco-BU tracking IDs in our Cisco.com web data, we will map a Cisco login ID back to a web cookie ID to fill in some of the web attribution gaps we see on sites like renew.cisco.com after a user logs in with SSO.
Once we had established that level of data threading, we could develop our own Sankey diagrams using our existing Tableau platform for Customer Journey Analytics. Additionally, leveraging our existing tech stack helps limit the number of reporting platforms used to ensure better metrics consistency and easier maintenance.
Use Case #4: Easily integrate data science to branch and personalize the customer journey.
We wanted to explore how we can take the output of a data science model and pivot a journey to provide a more personalized, guided experience for that customer. For instance, let’s look at our customer’s renewal journey. Today, they receive a four-touchpoint journey reminding them to renew. Customers can also open a chat or have a representative call or email them for additional support. Ultimately, the journey is the same for a customer regardless of their likelihood to renew. We have, however, a churn risk model that could be leveraged to modify the experience based on high, medium, or low risk of churn.
So, if a customer with an upcoming renewal had a high risk of churn, we could trigger a prescriptive action to escalate to a human for engagement, and we could also personalize the email with a more urgent message for that user. Whereas a customer with a low risk for churn could have an upsell opportunity weaved into their notification or we could route the low-risk customers into advocacy campaigns.
The goals of this use case were primarily:
1. Leverage the output of a data science model to personalize the customer’s experience
2. Pivot experiences from digital to human escalation based on data triggers.
3. Provide context to help customer agents understand the opportunity and better engage the customer to drive the renewal.
The Challenge
This was actually a rather natural fit for an orchestration platform. The challenge we entered here was the data refresh timing. We needed to refresh the renewals data to be processed by the churn risk model and align that with the timing of the triggered email journeys. Our renewals data was refreshed at the beginning of every month, but we hold our sends until the end of the month to allow our partners some time to review and modify their customers’ data prior to sending. Our orchestration platform would only process new, incremental data and overwrite based on a pre-identified primary key (this allowed for better system processing to not just overwrite all data with every refresh).
To get around this issue, our vendor would create a brand new view of the table prior to our triggered send so that all data was newly processed (not just any new or updated records). Not only did this create a vendor dependency for our journeys, but it also introduced potential quality assurance issues by requiring a pre-launch update of our data table sources for our production journeys.
Our Next Step Forward
One question we kept asking ourselves as we struggled to make this use case work with the orchestration platform—were we overcomplicating things? The two orchestration platform outputs of our attrition model use case were to:
1. Customize the journey content for a user depending on their risk of attrition.
2. Create a human touchpoint in our digital renewal journey for those with a high attrition risk.
For number one, we could actually achieve that using dynamic content modules within SalesForce Marketing Cloud if we simply added a “risk of attrition” field to our renewals data extension and created dynamic content modules for low, medium, and high risk of attrition values. Done!
For number two, doesn’t that sound sort of familiar? It should! It’s the same problem we wanted to solve in our first use case for prescriptive calls to action. Because we already worked to create a new architecture for scaling our recommended actions across multiple channels and audiences, we could work to add a branch for an “attrition risk” alert to be sent to our Cisco Renewals Managers and partners based on our data science model. A feedback loop could even be added to collect data on why a customer may not choose to renew after this human connection is made.
Finding Success
At the end of our one-year pilot, we had been forced to think about the tactics to achieve our goals very differently. Yes, we had deemed the pilot a failure – but how do we fail forward? As we encountered each challenge, we took a step back and evaluated what we learned and how we could use that to achieve our goals.
Ultimately, we figured out new ways to leverage our existing systems to not only achieve our core goals but also enable us to have end-to -end visibility of our code so we can set up the processing, refreshes, and connections exactly how our business requires.
Now – we’re applying each of these learnings. We are rolling out our core use cases as capabilities in our existing architecture, building an orchestration inventory that can be leveraged across the company – a giant step towards success for us and for our customers’ experience. The outcome was not what we expected, but each step of the process helped propel us toward the right solutions.
Source: cisco.com