Talking to your Network
Embarking on my journey as a network engineer nearly two decades ago, I was among the early adopters who recognized the transformative potential of network automation. In 2015, after attending
Cisco Live in San Diego, I gained a new appreciation of the realm of the possible. Leveraging tools like
Ansible and
Cisco pyATS, I began to streamline processes and enhance efficiencies within network operations, setting a foundation for what would become a career-long pursuit of innovation. This initial foray into automation was not just about simplifying repetitive tasks; it was about envisioning a future where networks could be more resilient, adaptable, and intelligent. As I navigated through the complexities of network systems, these technologies became indispensable allies, helping me to not only manage but also to anticipate the needs of increasingly sophisticated networks.
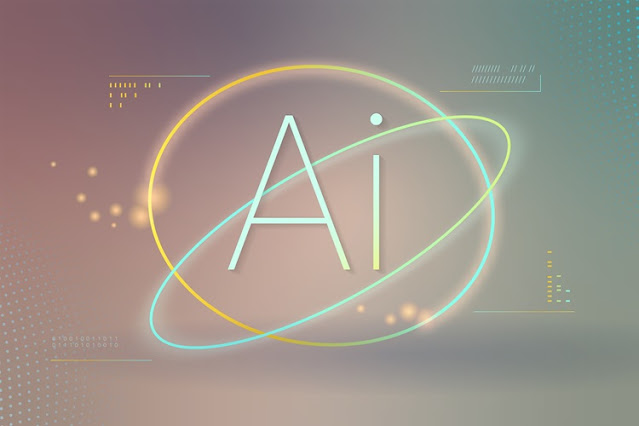
In recent years, my exploration has taken a pivotal turn with the advent of generative AI, marking a new chapter in the story of network automation. The integration of artificial intelligence into network operations has opened up unprecedented possibilities, allowing for even greater levels of efficiency, predictive analysis, and decision-making capabilities. This blog, accompanying the CiscoU Tutorial, delves into the cutting-edge intersection of AI and network automation, highlighting my experiences with Docker, LangChain, Streamlit, and, of course, Cisco pyATS. It’s a reflection on how the landscape of network engineering is being reshaped by AI, transforming not just how we manage networks, but how we envision their growth and potential in the digital age. Through this narrative, I aim to share insights and practical knowledge on harnessing the power of AI to augment the capabilities of network automation, offering a glimpse into the future of network operations.
In the spirit of modern software deployment practices, the solution I architected is encapsulated within Docker, a platform that packages an application and all its dependencies in a virtual container that can run on any Linux server. This encapsulation ensures that it works seamlessly in different computing environments. The heart of this dockerized solution lies within three key files: the Dockerfile, the startup script, and the docker-compose.yml.
The Dockerfile serves as the blueprint for building the application’s Docker image. It starts with a base image, ubuntu:latest, ensuring that all the operations have a solid foundation. From there, it outlines a series of commands that prepare the environment:
FROM ubuntu:latest
# Set the noninteractive frontend (useful for automated builds)
ARG DEBIAN_FRONTEND=noninteractive
# A series of RUN commands to install necessary packages
RUN apt-get update && apt-get install -y wget sudo ...
# Python, pip, and essential tools are installed
RUN apt-get install python3 -y && apt-get install python3-pip -y ...
# Specific Python packages are installed, including pyATS[full]
RUN pip install pyats[full]
# Other utilities like dos2unix for script compatibility adjustments
RUN sudo apt-get install dos2unix -y
# Installation of LangChain and related packages
RUN pip install -U langchain-openai langchain-community ...
# Install Streamlit, the web framework
RUN pip install streamlit
Each command is preceded by an echo statement that prints out the action being taken, which is incredibly helpful for debugging and understanding the build process as it happens.
The startup.sh script is a simple yet crucial component that dictates what happens when the Docker container starts:
cd streamlit_langchain_pyats
streamlit run chat_with_routing_table.py
It navigates into the directory containing the Streamlit app and starts the app using streamlit run. This is the command that actually gets our app up and running within the container.
Lastly, the docker-compose.yml file orchestrates the deployment of our Dockerized application. It defines the services, volumes, and networks to run our containerized application:
version: '3'
services:
streamlit_langchain_pyats:
image: [Docker Hub image]
container_name: streamlit_langchain_pyats
restart: always
build:
context: ./
dockerfile: ./Dockerfile
ports:
- "8501:8501"
This docker-compose.yml file makes it incredibly easy to manage the application lifecycle, from starting and stopping to rebuilding the application. It binds the host’s port 8501 to the container’s port 8501, which is the default port for Streamlit applications.
Together, these files create a robust framework that ensures the Streamlit application — enhanced with the AI capabilities of LangChain and the powerful testing features of Cisco pyATS — is containerized, making deployment and scaling consistent and efficient.
The journey into the realm of automated testing begins with the creation of the testbed.yaml file. This YAML file is not just a configuration file; it’s the cornerstone of our automated testing strategy. It contains all the essential information about the devices in our network: hostnames, IP addresses, device types, and credentials. But why is it so crucial? The testbed.yaml file serves as the single source of truth for the pyATS framework to understand the network it will be interacting with. It’s the map that guides the automation tools to the right devices, ensuring that our scripts don’t get lost in the vast sea of the network topology.
Sample testbed.yaml
---
devices:
cat8000v:
alias: "Sandbox Router"
type: "router"
os: "iosxe"
platform: Cat8000v
credentials:
default:
username: developer
password: C1sco12345
connections:
cli:
protocol: ssh
ip: 10.10.20.48
port: 22
arguments:
connection_timeout: 360
With our testbed defined, we then turn our attention to the _job file. This is the conductor of our automation orchestra, the control file that orchestrates the entire testing process. It loads the testbed and the Python test script into the pyATS framework, setting the stage for the execution of our automated tests. It tells pyATS not only what devices to test but also how to test them, and in what order. This level of control is indispensable for running complex test sequences across a range of network devices.
Sample _job.py pyATS Job
import os
from genie.testbed import load
def main(runtime):
# ----------------
# Load the testbed
# ----------------
if not runtime.testbed:
# If no testbed is provided, load the default one.
# Load default location of Testbed
testbedfile = os.path.join('testbed.yaml')
testbed = load(testbedfile)
else:
# Use the one provided
testbed = runtime.testbed
# Find the location of the script in relation to the job file
testscript = os.path.join(os.path.dirname(__file__), 'show_ip_route_langchain.py')
# run script
runtime.tasks.run(testscript=testscript, testbed=testbed)
Then comes the pièce de résistance, the Python test script — let’s call it capture_routing_table.py. This script embodies the intelligence of our automated testing process. It’s where we’ve distilled our network expertise into a series of commands and parsers that interact with the Cisco IOS XE devices to retrieve the routing table information. But it doesn’t stop there; this script is designed to capture the output and elegantly transform it into a JSON structure. Why JSON, you ask? Because JSON is the lingua franca for data interchange, making the output from our devices readily available for any number of downstream applications or interfaces that might need to consume it. In doing so, we’re not just automating a task; we’re future-proofing it.
Excerpt from the pyATS script
@aetest.test
def get_raw_config(self):
raw_json = self.device.parse("show ip route")
self.parsed_json = {"info": raw_json}
@aetest.test
def create_file(self):
with open('Show_IP_Route.json', 'w') as f:
f.write(json.dumps(self.parsed_json, indent=4, sort_keys=True))
By focusing solely on pyATS in this phase, we lay a strong foundation for network automation. The testbed.yaml file ensures that our script knows where to go, the _job file gives it the instructions on what to do, and the capture_routing_table.py script does the heavy lifting, turning raw data into structured knowledge. This approach streamlines our processes, making it possible to conduct comprehensive, repeatable, and reliable network testing at scale.
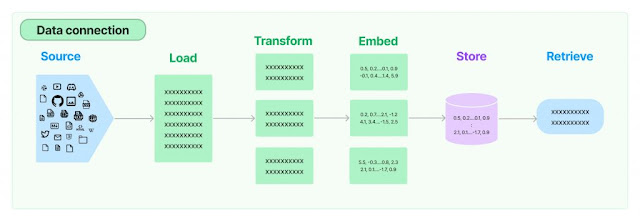
Enhancing AI Conversational Models with RAG and Network JSON: A Guide
In the ever-evolving field of AI, conversational models have come a long way. From simple rule-based systems to advanced neural networks, these models can now mimic human-like conversations with a remarkable degree of fluency. However, despite the leaps in generative capabilities, AI can sometimes stumble, providing answers that are nonsensical or “hallucinated” — a term used when AI produces information that isn’t grounded in reality. One way to mitigate this is by integrating Retrieval-Augmented Generation (RAG) into the AI pipeline, especially in conjunction with structured data sources like network JSON.
What is Retrieval-Augmented Generation (RAG)?
Retrieval-Augmented Generation is a cutting-edge technique in AI language processing that combines the best of two worlds: the generative power of models like GPT (Generative Pre-trained Transformer) and the precision of retrieval-based systems. Essentially, RAG enhances a language model’s responses by first consulting a database of information. The model retrieves relevant documents or data and then uses this context to inform its generated output.
The RAG Process
The process typically involves several key steps:
- Retrieval: When the model receives a query, it searches through a database to find relevant information.
- Augmentation: The retrieved information is then fed into the generative model as additional context.
- Generation: Armed with this context, the model generates a response that’s not only fluent but also factually grounded in the retrieved data.
The Role of Network JSON in RAG
Network JSON refers to structured data in the JSON (JavaScript Object Notation) format, often used in network communications. Integrating network JSON with RAG serves as a bridge between the generative model and the vast amounts of structured data available on networks. This integration can be critical for several reasons:
- Data-Driven Responses: By pulling in network JSON data, the AI can ground its responses in real, up-to-date information, reducing the risk of “hallucinations.”
- Enhanced Accuracy: Access to a wide array of structured data means the AI’s answers can be more accurate and informative.
- Contextual Relevance: RAG can use network JSON to understand the context better, leading to more relevant and precise answers.
Why Use RAG with Network JSON?
Let’s explore why one might choose to use RAG in tandem with network JSON through a simplified example using Python code:
- Source and Load: The AI model begins by sourcing data, which could be network JSON files containing information from various databases or the internet.
- Transform: The data might undergo a transformation to make it suitable for the AI to process — for example, splitting a large document into manageable chunks.
- Embed: Next, the system converts the transformed data into embeddings, which are numerical representations that encapsulate the semantic meaning of the text.
- Store: These embeddings are then stored in a retrievable format.
- Retrieve: When a new query arrives, the AI uses RAG to retrieve the most relevant embeddings to inform its response, thus ensuring that the answer is grounded in factual data.
By following these steps, the AI model can drastically improve the quality of the output, providing responses that are not only coherent but also factually correct and highly relevant to the user’s query.
class ChatWithRoutingTable:
def __init__(self):
self.conversation_history = []
self.load_text()
self.split_into_chunks()
self.store_in_chroma()
self.setup_conversation_memory()
self.setup_conversation_retrieval_chain()
def load_text(self):
self.loader = JSONLoader(
file_path='Show_IP_Route.json',
jq_schema=".info[]",
text_content=False
)
self.pages = self.loader.load_and_split()
def split_into_chunks(self):
# Create a text splitter
self.text_splitter = RecursiveCharacterTextSplitter(
chunk_size=1000,
chunk_overlap=100,
length_function=len,
)
self.docs = self.text_splitter.split_documents(self.pages)
def store_in_chroma(self):
embeddings = OpenAIEmbeddings()
self.vectordb = Chroma.from_documents(self.docs, embedding=embeddings)
self.vectordb.persist()
def setup_conversation_memory(self):
self.memory = ConversationBufferMemory(memory_key="chat_history", return_messages=True)
def setup_conversation_retrieval_chain(self):
self.qa = ConversationalRetrievalChain.from_llm(llm, self.vectordb.as_retriever(search_kwargs={"k": 10}), memory=self.memory)
def chat(self, question):
# Format the user's prompt and add it to the conversation history
user_prompt = f"User: {question}"
self.conversation_history.append({"text": user_prompt, "sender": "user"})
# Format the entire conversation history for context, excluding the current prompt
conversation_context = self.format_conversation_history(include_current=False)
# Concatenate the current question with conversation context
combined_input = f"Context: {conversation_context}\nQuestion: {question}"
# Generate a response using the ConversationalRetrievalChain
response = self.qa.invoke(combined_input)
# Extract the answer from the response
answer = response.get('answer', 'No answer found.')
# Format the AI's response
ai_response = f"Cisco IOS XE: {answer}"
self.conversation_history.append({"text": ai_response, "sender": "bot"})
# Update the Streamlit session state by appending new history with both user prompt and AI response
st.session_state['conversation_history'] += f"\n{user_prompt}\n{ai_response}"
# Return the formatted AI response for immediate display
return ai_response
Conclusion
The integration of RAG with network JSON is a powerful way to supercharge conversational AI. It leads to more accurate, reliable, and contextually aware interactions that users can trust. By leveraging the vast amounts of available structured data, AI models can step beyond the limitations of pure generation and towards a more informed and intelligent conversational experience.
Source: cisco.com